· 5 min read
Building a Data-Driven Organization? Here’s Why Data Quality Comes First
Building a data-driven culture starts with measuring data quality. See why data reliability matters.
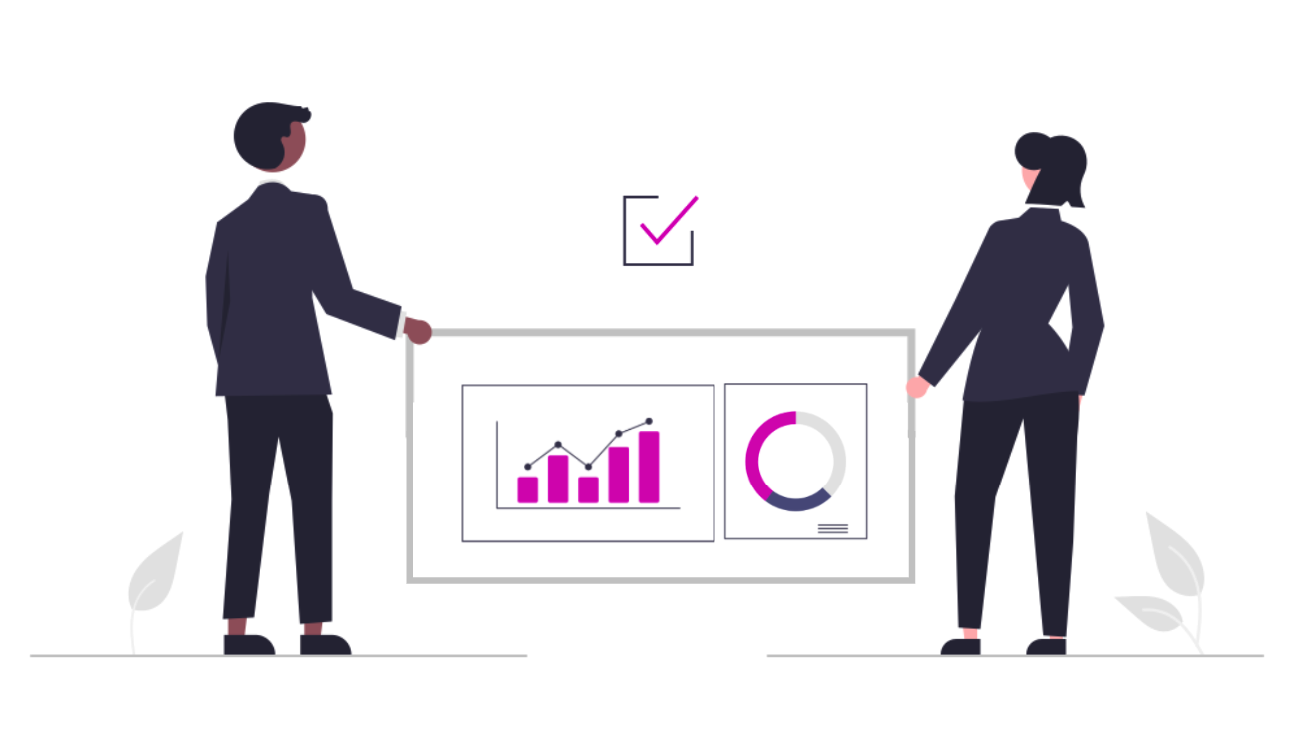
By: Oxana Urdaneta
Building a data-driven organization starts with a foundation of high-quality, trustworthy data. While data quality is often treated as a checkbox in data engineering processes, it’s far more than a technical requirement. In reality, data quality can make or break your organization’s data strategy and the value of your data investments. Many data teams focus on pipeline efficiency and ensuring seamless data delivery, while the measurement of data quality itself is sometimes overlooked.
In data engineering, there’s a well-known saying: “garbage in, garbage out.” While data teams can’t invent new or perfect data, they play a crucial role in transforming data so that business stakeholders can derive real value. When data reliability isn’t measured, it’s hard to convince the organization to base their decisions on data, and even the most advanced use cases may fail to build the necessary trust.
Data Quality Is Contextual, Not Generic
Data quality is deeply contextual, defined by how the data is used and what it needs to achieve within the organization. For example, a data set that’s “high quality” for a reporting dashboard may need entirely different metrics to be considered reliable for a machine learning algorithm. Similarly, what’s critical for one team may not be a priority for another.
Data leaders often work to influence business teams to embrace data, simplifying workflows and encouraging data-driven decisions as part of their daily operations. However, this mission relies on providing them with trustworthy data—data that’s reliable enough to influence high-stakes decisions and deliver measurable impact.
Becoming Data-Driven Means Measuring Reliability
A truly data-driven organization measures data reliability at every stage to ensure that business insights are built on a foundation of trusted data. Understanding the quality of incoming data and monitoring data health are critical steps for supporting the business effectively. While data issues often originate in upstream sources—like application teams or third-party vendors—it’s up to data teams to measure these issues, gauge their impact, and work with those responsible to resolve them.
For example, Konstellation’s Table Reliability Score provides an out-of-the-box metric to quickly assess data reliability across tables. By tracking key metrics like data freshness, quality, and stability, Konstellation offers a comprehensive view of data health, allowing teams to measure reliability across their tables efficiently, and in a standardized way.
While data quality requirements may vary based on business impact and data use cases, prioritizing measurement is key. Some use cases can work with data that is “directionally correct,” while others need rigorous precision. Either way, maintaining data quality standards across all levels builds the foundation for an organization that is genuinely data-driven.
The Levels of Data Quality Detection: Why Ownership Matters
Effective data quality management requires identifying and addressing issues as early as possible. The sooner an issue is detected in the data pipeline, the fewer disruptions it causes for internal or external teams who depend on that data. Ideally, all issues are caught and resolved by the data engineering teams before they reach other users. However, when issues do slip through, they create higher risk and impact based on who is accessing the unreliable data. Let’s review these levels:
- Level 1: Data Engineering Team
The data engineering team is the first line of defense and should ideally be the first to know and address any data quality issues. When data engineers are equipped with quality monitoring tools, they can catch anomalies early, before they affect other teams or external customers. This reduces downstream impact and allows the data team to maintain higher standards. - Level 2: Analytics or Data Science Teams
The analytics and data science teams, as key partners within the data function, rely on accurate data to build models and generate insights. When quality issues slip through, they are forced to spend time fixing or cleaning the data before they can extract insights or implement models, slowing down decision-making processes. While it’s not ideal for them to catch these issues, they serve as an important internal partner in identifying areas where data quality could be improved. - Level 3: Internal Business Teams
When internal business teams are the ones to find data quality issues, it directly affects their confidence in the data team’s work. Business users typically have a strong understanding of what the data should represent, so inconsistencies are often alarming and can raise doubts.Although these teams can advocate for improvements, their ability to make informed decisions suffers when data quality is compromised. Ideally, they shouldn’t be the ones detecting these issues, as this not only disrupts their workflows but also affects the data team’s reputation across the organization. - Level 4: External Customers
If data quality issues reach external customers, the stakes are highest. Customers rely on accurate, timely data, and any inconsistencies can damage trust and credibility for the whole organization. Data quality issues at this level lead customers to question whether the organization can reliably deliver on its promises and SLAs, creating lasting harm to the brand and the customer relationship.
Why Data Quality Ownership Starts with the Data Team
Taking ownership of data quality does more than prevent errors; it establishes a foundation of trust and confidence throughout the organization. When data quality is a priority, data teams can provide reliable data products that make it easy for both internal stakeholders and external customers to depend on, leading to truly data-driven outcomes. This commitment to quality offers peace of mind and a stronger foundation for decision-making.
By focusing on measurement, continuous improvement, and collaborative problem-solving with upstream and external sources, data teams can transform data quality from a checklist item into a strategic asset. After all, if data quality isn’t prioritized, how can the rest of the organization trust it to drive meaningful outcomes?
Conclusion
Building a data-driven culture starts with a commitment to data quality. By recognizing the levels at which data quality issues can arise and proactively addressing them, data teams can ensure that their organization’s data strategy is built on a foundation of trust and reliability. With robust data quality practices, organizations can move confidently towards becoming truly data-driven.