· 7 min read
Exploring Data Mesh - Benefits, Challenges, and Observability
Explore the benefits and challenges of adopting a data mesh architecture and its impact on your data strategy.
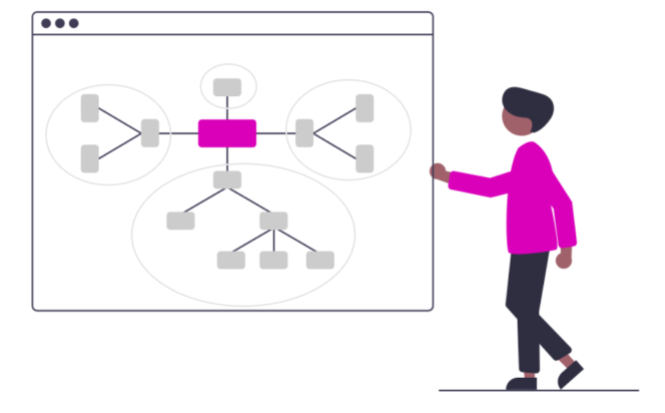
By: Oxana Urdaneta
In the rapidly evolving landscape of data management, the concept of a data mesh has emerged as a game-changer. As organizations deal with the complexities of big data, the need for scalable, flexible, and resilient data architectures has never been more pressing. Data mesh offers a promising solution, but like any architectural shift, it has its own benefits and challenges. This post will delve into what a data mesh architecture is, why it has gained traction, and how data observability fits into this paradigm.
What is Data Mesh?
Traditional data architectures often rely on centralized data lakes or warehouses, bringing data from various sources together for processing and analysis. While this approach has been practical, it can lead to bottlenecks, scalability issues, and challenges in managing the volume and complexity of data while addressing the organization’s different data use cases.
A data mesh consists of a decentralized model in which data is treated as a product owned by specific domains within an organization. These domains typically correspond to key business units or functional areas within an organization, such as Sales, Marketing, Product, Customer Experience, etc. Each domain acts as a mini data team, responsible for its data products, ensuring that data is accessible, trustworthy, and aligned with the business’s needs. This approach is built on four fundamental principles:
- Decentralized Data Ownership: Each domain owns its data, taking full responsibility for its quality, governance, and availability.
- Data as a Product: Data is treated as a product, with domain teams providing reliable, discoverable, and accessible data to other teams within the organization.
- Self-Serve Data Infrastructure: Teams are empowered with the tools and platforms to manage their data independently without relying on a centralized data team.
- Federated Governance: While data ownership is decentralized, governance remains a shared responsibility across the organization, ensuring compliance and standardization without slowing down innovation.
A key takeaway from this shift is that decentralized data ownership isn’t just about distributing existing processes and enabling teams to be fully accountable for their data lifecycle. Additionally, treating data as a product requires a mindset shift where domain data producers consider data consumers as customers, ensuring that data products are reliable, discoverable, and usable. Even though these four principles are fundamental for a complete data mesh architecture, it’s often easier to get started with the first two (decentralization and data-as-a-product) and gradually implement the latter two (self-serve infrastructure and federated governance) over time.
Why Should Organizations Consider Data Mesh?
The shift towards data mesh is primarily driven by the challenges posed by traditional centralized data architectures. As organizations grow, their data becomes more complex, leading to bottlenecks, slower time-to-insight, and increasing friction between data producers and consumers.
Here’s why data mesh is gaining popularity:
- Scalability: Traditional data architectures often struggle to scale with data’s growing volume and variety. Data mesh allows organizations to scale their data operations more efficiently by decentralizing ownership and management.
- Agility: By giving domains ownership of their data, organizations can respond more quickly to changing business needs. This agility is critical in today’s fast-paced environment, where timely insights can provide a competitive edge.
- Improved Data Quality: With each domain responsible for its data, quality becomes a priority. Domains work closer to their stakeholders, understand the domain use cases better, and are motivated to maintain high standards to ensure their data is usable and reliable for others in the organization.
- Better Alignment with Business Needs: Data mesh aligns data management with the specific needs of each business unit. This leads to data products that are more relevant, actionable, and valuable to the organization as a whole.
When Should You Consider Data Mesh?
While data mesh offers significant advantages, it’s not a one-size-fits-all solution. Organizations should consider several factors before making the shift:
- Organizational Structure: Data mesh works best in organizations already structured around autonomous business domains. If your organization is highly centralized, transitioning to a data mesh may require a significant cultural and operational shift.
- Data Maturity: Organizations with mature data practices and robust governance frameworks are better positioned to succeed with data mesh. Without these foundations, decentralization can lead to data silos and inconsistencies.
- Complexity and Scale: If your organization deals with complex, high-volume data that spans multiple business domains, a data mesh could provide the scalability and flexibility you need. However, for smaller, less complex data environments, the overhead of implementing a data mesh may outweigh the benefits.
Overcoming Common Challenges When Implementing a Data Mesh Architecture
While the data mesh architecture offers numerous advantages, it also comes with its own set of challenges that organizations must carefully consider before adoption:
- Complexity of Implementation: Moving to a data mesh model involves a significant technological and organizational culture shift. You must rethink team roles, data management practices, and governance structures. This transition can be complex and requires clear communication and a well-defined action plan to manage resistance and ensure a smooth transition.
- Risk of Data Silos: Even though data mesh aims to break down centralized data silos, new silos can emerge if not carefully managed. To avoid this, ensure that all domains adhere to consistent data standards and collaborate effectively. Strong governance and regular cross-domain coordination are key to maintaining a unified data landscape.
- Governance and Standardization: Balancing autonomy with compliance is challenging in a decentralized model. Implement robust data contracts between domain teams and oversight mechanisms to ensure all domains follow organizational standards while retaining independence. This will help maintain consistency and prevent fragmented practices as the organization grows.
- Increased Operational Overhead: Managing independent domains can lead to higher operational demands. Each domain needs data pipelines, monitoring, and incident resolution processes. Investing in advanced monitoring and observability tools can help manage this complexity and ensure domains meet their performance targets.
- Managing Inter-Dependencies: With decentralized pipelines, inter-dependencies between domains need careful management. Use modular designs, APIs, and clear data contracts to maintain compatibility and minimize disruptions. This approach helps ensure that changes in one domain do not negatively impact others.
The Role of Data Observability in Data Mesh
Data observability resolves the complexities of standardizing quality and governance while enabling decentralization and growth.
Data observability provides comprehensive visibility into data pipelines across various domains in a data mesh architecture. This ensures that the organization maintains a holistic and standardized view of its data landscape even as data ownership becomes distributed.
Data observability supports proactive monitoring and anomaly detection by leveraging real-time analytics and machine learning to identify potential issues before they escalate. This early detection helps prevent disruptions and maintains high data reliability. Tracking critical data quality metrics (such as data freshness, accuracy, and completeness) offers valuable insights into data health and supports timely issue resolution.
Additionally, data observability is critical to supporting federated governance. While domains operate autonomously, it ensures that they still adhere to organizational standards and compliance requirements, achieving a balanced approach. This flexibility is essential in a data mesh, where the architecture must grow without compromising data quality or reliability.
As organizations scale and introduce new domains, data observability tools scale with them, maintaining data quality and fostering a culture of transparency and continuous improvement. These tools enhance overall data management practices and support a data-driven culture within the organization.
Conclusion: Empowering Your Decision
Data mesh represents a significant shift in how organizations manage and utilize their data. By decentralizing data ownership and treating data as a product, it offers a path to greater scalability, agility, and alignment with business needs. However, it’s not without its challenges, and organizations must carefully consider whether this architecture aligns with their structure, maturity, and data complexity.